Discover AI's impact on clinical trials, highlighting efficiency gains and regulatory hurdles.
AI in Clinical Trials
As biotech, pharma, and medical device companies seek to bring new drugs and technologies to market in more efficient and effective means, AI is at the forefront and playing a critical role in clinical trials. Clinical trials are time-consuming and costly, and sponsors are seeking ways to streamline clinical trial processes. AI is transforming all facets of clinical research from early drug development to clinical trials to post market surveillance.
The number of clinical trials involving AI is rapidly increasing. The US Food and Drug Administration (FDA) has seen a substantial increase in the drug applications utilizing AI, with more than 100 submissions reported in 2021.
What is AI
Artificial intelligence (AI) is a branch of computer science, statistics, and engineering that utilizes algorithms or predictive modeling to allow machines to perform tasks and exhibit behaviors such as learning, making decisions, and making predictions. Machine learning (ML) is considered a subset of AI that allows models to be developed by training algorithms through the analysis of data, without models being explicitly programmed.
AI, IRBs, and Human Subjects Research
Most AI research falls outside of regulatory oversight because 1) the data involved are often collected, owned, and used by commercial entities, which are largely unregulated, 2) research depends on the collection of massive amounts of data from social media, apps, internet browsing histories, wearable devices, and electronic health records and most of the data being analyzed is either deidentified or already available within public domain, and exempt from IRB review, and 3) while there may be risks to people whose data is included within large data sets that are used to train algorithms—reidentification being the most obvious—the risks are considered low.
However, it’s critical for Institutional Review Boards (IRBs) to understand when protocols presented to the IRB are human subject research and thus regulated. Research Studies have been incorrectly presented to IRBs as software development projects or secondary-use data projects and IRBs have inaccurately given non-human subject research determinations and exempt determinations. AI/ML based software, when intended to treat, diagnose, cure, mitigate, or prevent disease or other conditions, are still considered medical devices and are regulated by the FDA as Software as a Medical Device (SaMD).
Is Standard IRB Review Adequate
The IRB must be able to evaluate how AI will impact the IRBs risk to benefit analysis. They must consider any potential harms related to the validity and bias of models used to train AI, in addition to how the privacy of research participants will be protected. Additionally, IRBs must ensure the consent form includes the use of AI as part of the research if AI is being used to make decisions about participant care as well as any secondary data use following the study’s conclusion.
Because there are currently no existing regulations or regulatory guidance pertaining to the IRB’s review of clinical trials involving AI, IRBs are forced to use existing ethical standards as well as utilizing the same criteria for IRB approval that’s currently used for all other research, such as risk minimization, risks reasonable in relation to anticipated benefits, equitable selection of subjects, informed consent, data safety monitoring, privacy protections and data confidentiality, and protections for vulnerable populations. However, are the current guidance and standards enough to provide appropriate protections for research participants in trials where AI systems are employed? Or should there be additional oversight requirements for AI trials?
Addressing Ethical Concerns with AI Research
AI in research is typically used to develop tools that will replace human decision making. This involves the collection and farming of large amounts of data that is then used to train an algorithm to make decisions or predictions. The algorithm is then tested and validated based on the accuracy of the decisions and predictions.
Ethical concerns with AI research include: consent to use data, algorithmic fairness and biases, safety and transparency, and data privacy and identifiability.
Suggested Process for Informed Consent:
- Inform participants of the AI’s use in the trial when its use is part of their care or wellbeing, along with the data the system will access and use
- Explain how the AI tool functions
- Include a description of what the AI will do with the data it receives as a result of the research activities
- Inform the participant when the scope of the AI tool’s ability to utilize or re-access data that it has been previously provided is not fully known or understood
- Inform participants if and when their data cannot be removed from the AI tool
- Clearly explain limitations of privacy and confidentiality, i.e., third party vendors Google, Amazon, and the possibility of re-identification, if applicable
- Describe how the participants data is used, stored, shared, destroyed or not destroyed, de-identified or not de-identified, during and after the trial
Data Bias: If the initial programmed input data is biased, it could lead to inaccurate results or decisions based on biases. The algorithm must train on unbiased data and then be tested and validated for accuracy prior to deployment in a real-world setting.
Safety: Testing technology in restricted real-world scenarios is imperative for identifying and assessing risks and establishing effective monitoring.
Transparency: Clearly identifying and explaining the source and characteristics of data and methods used to train the model.
- Explainability: A technique that enables human users to understand and effectively manage AI. When IRBs understand the AI explainability they are able to adequately fulfill their responsibility of assessing risk-benefit ratio. The process is comprehendible and validation is accepted. Explainability AI fosters transparency and trust.
- Blackbox: Some AI systems are extremely complex or may not be fully transparent for proprietary reasons and humans are unable to understand how a trained and validated algorithm arrives at the decisions or predictions that it does. The lack of explainability of the system’s decision-making is hard to identify, correct, or repair any aspect that might be incorrect in how the tool is applied. This poses transparency issues and results are difficult to justify and fully validate with certainty.
Data Privacy & Identifiability: Patient data must remain secure and be protected from unauthorized access, use, manipulation, and disclosure. Researchers should take additional measures to ensure any stored data is encrypted as well as using strong authentication methods to protect access to sensitive information. If it is possible that an AI system has the ability to create indirect identifiers within a dataset, caution should be taken. It is highly advisable to limit an AI system’s access to demographic information and other data that could potentially reidentify an individual.
Specific Considerations for AI Research
In an effort to promote principles of trustworthy AI, IRBs should consider obtaining the following information from researchers to aide in their review of protocols developing AI tools**:
- A description of how the data used to train/validate the AI tool is representative of the population the algorithm is designed to impact. Describe the underlying population of interest and the sample that was used to train the model.
- A description of data wrangling tools and analytical controls that will be used to actively mitigate the potential effects of statistical bias and other misattributions of cause in or on the data.
- A description of the plan to ensure the data used to train the AI tool will be used to perform effective AI-decision making, as well as the metrics that will be used to evaluate the effectiveness.
- Identify and list the different sources of data used to train or validate any AI tool(s) and the way that the sample is constructed (e.g., with nationally representative sample weights.)
- Whether the AI algorithm is intended to be used for commercial profit.
- Identify any big data repositories that primarily store data used to train the AI algorithm.
- Describe plans to ensure that the privacy and security of data are maintained during and after the research process, particularly, through the application of AI.
- Describe any decisions or recommendations that the AI tools would be making for human subjects, and how these conclusions are reached and any limitations to them.
- Describe if research participants are specifically being informed of the use of the tool through the informed consent process.
- Will research participants be impacted by decisions made by any AI tools? If yes, describe any risks associated with the application of AI to the study or research participants, including the potential impact severity on study participants. Categorize the type of decision-making by AI:
- AI is used to help inform human decisions.
- AI drives decisions with human oversight.
- AI is fully autonomous (i.e., no oversight).
Improving IRBs Understanding and Review of AI Research
Considerations for IRB review of AI research is quite different from what IRBs are used to seeing. To better position IRBs to provide a meaningful review that ensures the regulatory and ethical objectives are appropriate in assessing risk benefit, and to ensure protocols are designed with appropriate protections for research participants, IRBs should invest in the following:
Education and Training on AI in human subject’s research is imperative.
Include an AI data expert or a researcher with experience in AI on the IRB or as a consultant available as needed by the IRB
Incorporate the above ’Specific Considerations for AI Research’ adapted to fit the IRBs needs into submission requirements.
Utilize IRB reviewer checklists to aid in determining which studies are developing AI human subjects research versus studies using AI but not involving human subjects.
Collaboration between all stakeholders – IRBs, researchers, and industry is paramount.
Conclusion
As AI continues to transform clinical trials, and with the lack of existing regulatory guidance surrounding its use, it is important to partner with an organization that has an understanding in AI and IRBs, and who can provide researchers and sponsors with clear guidance on studies with AI components.
Priscilla is an FDAQRC consultant that has more than 25 years’ experience in clinical trials, specializes in regulatory compliance and IRB administration, and is a human subject protection SME. She is passionate about the administration of ethical, compliant, and quality clinical trials, operational efficiency, and service excellence. Priscilla brings a wealth of expertise in good clinical practices, GCP audits, IRB operations, quality review of regulatory documentation, and compliance, in all phases of clinical trials and various therapeutic areas, including Phase I and oncology. As a business leader, she has led companies in attaining AAHRPP accreditation, successful regulatory inspections, and sponsor audits.
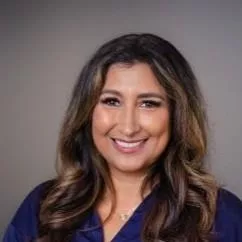
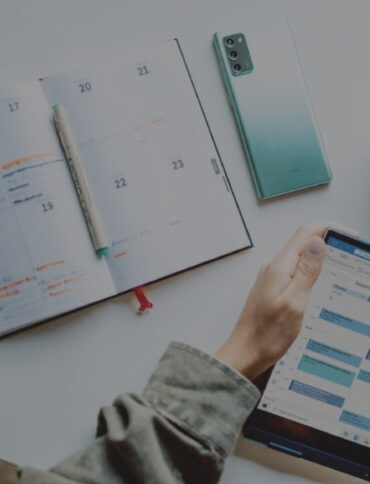
Tips For Preparing & Hosting a Successful Audit
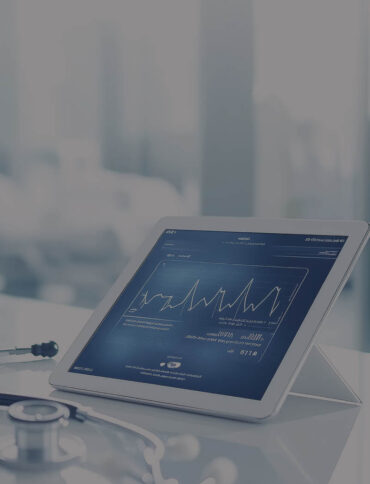
Regulating AI in Clinical Trials: What ICH E6(R3) Does (and Doesn’t) Say
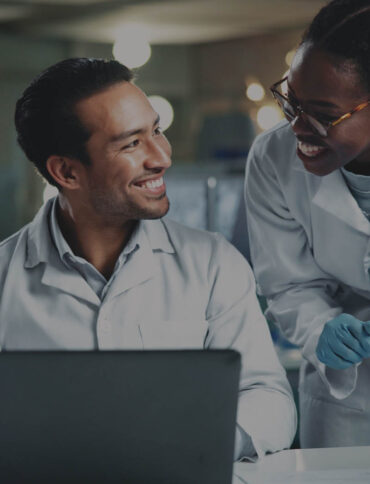
Explores the Differences Between Auditors and Inspectors in the Clinical Research Industry
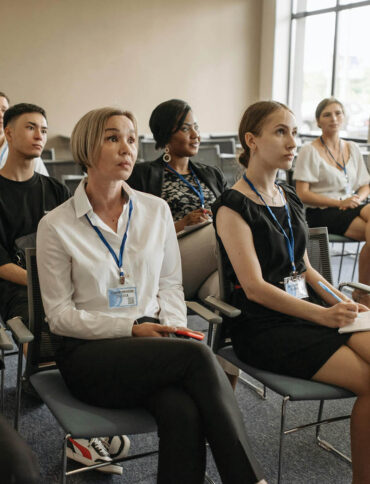
Competency Based Programs Contribute to Early Clinical Research Professional’s Training
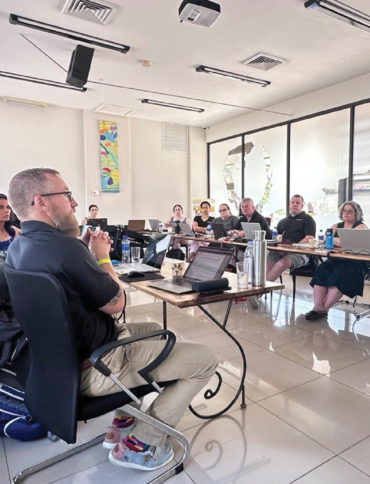
15 Years of Excellence: FDAQRC's Journey and Future Vision
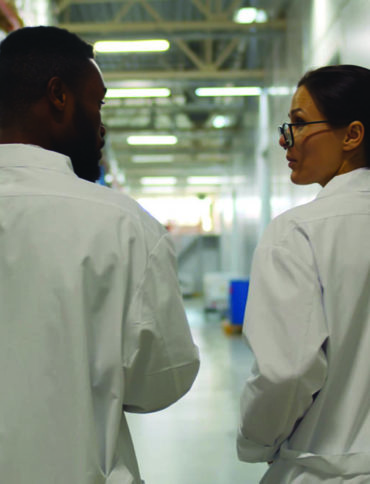
Tips for a Positive Outcome While Performing a Challenging Audit
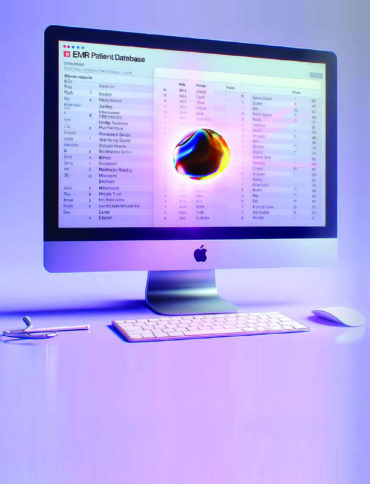
How To Find Qualified Patients for Your Clinical Trials
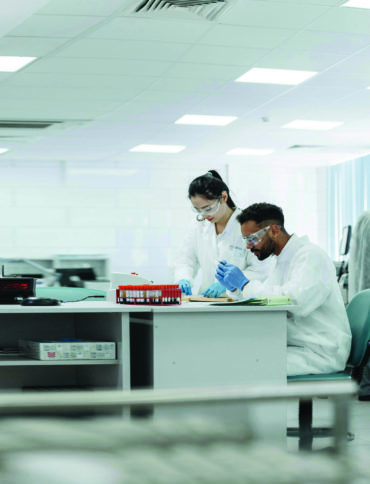